Mobile app personalization features are transforming user experiences, moving beyond simple customization to a deep understanding of individual needs. This intricate process leverages user data to deliver tailored content, recommendations, and even dynamic interfaces, creating engaging and relevant interactions.
From targeted recommendations to personalized notifications, the possibilities are vast. This exploration delves into the core concepts, practical implementation strategies, and the ethical considerations surrounding this powerful technology.
Defining Mobile App Personalization

Mobile app personalization is all about tailoring the app experience to each user’s unique needs and preferences. It’s not just about slapping a user’s name on a screen; it’s about understanding their behaviors and using that knowledge to deliver relevant content, features, and recommendations. The goal is to create a more engaging, efficient, and ultimately satisfying experience for the user, leading to higher user retention and increased app usage.
Personalization goes beyond simply customizing the app’s look and feel. Instead, it dynamically adjusts the app’s functionality and content based on individual user data. This results in a more relevant and efficient experience, in contrast to a one-size-fits-all approach. Think of it as a conversation between the app and the user, where the app learns from each interaction to offer a better, more personalized conversation.
Core Principles of Personalization
Personalization in mobile apps hinges on several key principles. It requires understanding user needs, preferences, and behaviors. It uses this understanding to deliver relevant content, features, and experiences. Crucially, personalization must be transparent and ethical, respecting user privacy and data security. Furthermore, it should not be intrusive, maintaining a balance between offering value and avoiding user frustration.
Personalization vs. Customization
Personalization differs from customization. Customization allows users to adjust the app’s appearance and some basic features, like themes or notification settings. Personalization, however, goes deeper, adjusting the app’s content and functionality based on user behavior and preferences. Think of customization as a user adjusting the app’s thermostat, while personalization is the app learning the user’s preferred temperature and adjusting the thermostat automatically.
Types of User Data for Personalization
Several types of user data contribute to a personalized mobile app experience. Demographics, such as age, gender, and location, provide a broad understanding of the user. Usage patterns, including frequency of app use, specific features used, and time spent on different sections, offer a deeper understanding of individual preferences. Purchase history, if applicable, reveals specific interests and buying behaviors. Finally, user feedback, directly or indirectly provided, allows the app to further refine its understanding of the user and tailor experiences accordingly.
Benefits and Drawbacks of Personalization
Benefits | Drawbacks |
---|---|
Enhanced User Engagement: Personalized recommendations and content increase user interest and encourage continued app usage. | Potential for Privacy Concerns: Collecting and utilizing user data necessitates careful consideration of privacy implications. |
Increased Conversion Rates: Relevant product suggestions and targeted promotions can lead to higher conversion rates for in-app purchases. | Data Security Risks: Storing and processing sensitive user data necessitates robust security measures to protect against breaches. |
Improved User Experience: Tailored content and features make the app feel more intuitive and user-friendly. | Potential for Bias in Algorithms: Personalization algorithms can reflect existing biases in the data they’re trained on, potentially leading to unfair or discriminatory outcomes. |
Reduced User Effort: Personalized recommendations and features can reduce the user’s effort to find relevant information or products. | Over-Personalization and Intrusiveness: If personalization becomes excessive or intrusive, it can negatively impact the user experience. |
Increased App Retention: Users are more likely to stick with an app that feels tailored to their needs and preferences. | Algorithm Complexity: Building and maintaining sophisticated personalization algorithms can be complex and resource-intensive. |
Types of Personalization Features
Mobile app personalization isn’t just about slapping a user’s name on a screen; it’s about tailoring the entire experience to their individual needs and preferences. This involves a range of features that go beyond basic user profiles. Different types of personalization cater to diverse user behaviors and desires, leading to improved user engagement and satisfaction.
Targeted Recommendations
Personalized recommendations leverage user data to suggest relevant items, content, or actions. This can include product recommendations in e-commerce apps, music suggestions in streaming services, or even content recommendations in news apps. The effectiveness of these recommendations hinges on the accuracy and depth of the data used to predict user preferences.
- These recommendations are often based on past interactions, purchase history, or similar user behaviors. For instance, if a user frequently purchases a specific type of clothing, the app might recommend similar items or styles.
- Sophisticated algorithms analyze vast amounts of data to understand complex patterns and provide accurate and timely recommendations. These algorithms are constantly evolving to improve prediction accuracy.
- Examples include Spotify’s personalized music playlists, Netflix’s movie and TV show suggestions, and Amazon’s product recommendations.
Tailored Content
This approach delivers content specifically relevant to individual users. This might involve displaying different articles, news feeds, or promotional offers based on a user’s interests or location. The content is dynamic, adjusting to the user’s preferences and evolving as they interact with the app.
- A news app might tailor its headlines to a user’s preferred topics, providing a more engaging and informative experience.
- E-learning apps can adapt course materials based on a user’s learning pace and comprehension, leading to more efficient and effective learning outcomes.
- For example, a user interested in fitness might see articles about workout routines, while a user interested in finance might see articles about investment strategies.
Dynamic UI Elements
Personalization extends to the app’s user interface. Dynamic UI elements adjust based on user behavior and preferences. This includes changing colors, layouts, or even the overall presentation of the app. A visually appealing and intuitive interface is a key element of user satisfaction.
- The app’s layout might change based on the user’s preferred language or reading style.
- Navigation menus can be reorganized to reflect the user’s frequent actions, making the app more accessible and efficient.
- For instance, a social media app might display more posts from friends who frequently engage in conversations.
Comparison of Personalization Features
Feature Type | Functionality | Strengths | Limitations |
---|---|---|---|
Targeted Recommendations | Predictive suggestions based on user behavior | Improved user engagement, increased conversion rates | Requires large datasets, potential for irrelevant suggestions |
Tailored Content | Dynamic content delivery based on user preferences | Enhanced user experience, improved information relevance | Data privacy concerns, potential for filter bubbles |
Dynamic UI Elements | Adaptive interface based on user behavior | Increased user efficiency, improved visual appeal | Potential for complexity, difficulty in implementation |
Implementing Personalization Features
Personalization in mobile apps isn’t just a nice-to-have; it’s a key driver of user engagement and retention. Implementing these features effectively requires a deep understanding of the technical aspects, data handling, and the role of algorithms. This section dives into the practicalities of building personalized experiences.
Implementing personalization requires a strategic approach that goes beyond simply slapping on a recommendation engine. It demands careful consideration of data collection, processing, algorithm selection, and integration into the existing app architecture. Successful personalization projects prioritize user privacy, ensuring data is handled responsibly and ethically.
Technical Aspects of Implementation
Implementing personalization features necessitates understanding the technical infrastructure of your app. This includes the data storage methods, the communication protocols, and the overall architecture. Different approaches to personalization demand different technological solutions. For instance, real-time personalization might need a different setup than batch-based personalization.
Data Collection and Processing
Effective personalization hinges on collecting and processing the right data. Different data collection methods are suitable for different personalization goals.
- User Activity Data: This includes app usage patterns, frequency of use, interaction with specific features, and time spent on different screens. This data is crucial for understanding user preferences and behaviors.
- User Profile Data: Demographic information, purchase history, and preferences gathered from user profiles provide a comprehensive view of user characteristics.
- External Data Sources: Leveraging external data sources like weather information, events, and trending topics can enrich the personalization experience. However, careful consideration of data privacy and the ethical implications of using external data is essential.
Data processing involves cleaning, transforming, and structuring collected data. This preparation is essential for algorithms to accurately identify patterns and preferences. Tools like Apache Spark and Hadoop are often used for large-scale data processing. Robust data pipelines are crucial for consistent data flow and quality.
Role of Algorithms and Machine Learning
Algorithms are the engines driving personalization. Machine learning plays a pivotal role in developing these algorithms.
- Recommendation Engines: These engines use algorithms to predict what users might like based on their past behavior and preferences. A common example is recommending products a user might be interested in based on their past purchases or browsing history.
- Content Filtering: Algorithms can filter content based on user preferences. For example, a news app might filter articles based on the topics the user has shown interest in.
- Clustering: Grouping users with similar characteristics allows for targeted marketing and content delivery.
Machine learning models, like collaborative filtering and content-based filtering, are essential for creating effective recommendations. Choosing the right algorithm depends on the specific personalization goals and the type of data available.
Integrating a Recommendation Engine
A step-by-step procedure for integrating a recommendation engine is:
- Data Preparation: Ensure your data is clean, structured, and ready for the recommendation engine. This involves cleaning any errors, converting data types to suitable formats, and handling missing values.
- Algorithm Selection: Choose a recommendation engine algorithm that aligns with your app’s needs and data characteristics. Consider collaborative filtering or content-based filtering.
- Engine Integration: Integrate the chosen engine into your app’s architecture. This might involve creating APIs or using existing libraries.
- User Interface (UI) Integration: Display recommendations in a user-friendly way within the app. Consider the placement and presentation of the recommendations.
- Testing and Refinement: Thoroughly test the recommendations to ensure they are relevant and engaging for users. Iteratively refine the engine based on user feedback and performance metrics.
User Experience and Personalization
Personalization in mobile apps isn’t just about fancy features; it’s about crafting experiences that feel tailored to each user. A well-personalized app can significantly enhance engagement, satisfaction, and ultimately, user loyalty. This section dives into how to design and implement personalized experiences that resonate with users while respecting their privacy.
Personalized experiences go beyond just remembering user preferences. They’re about anticipating needs and offering relevant content, features, and recommendations. A truly personalized app feels intuitive and effortless, leading to a more positive user journey.
Impact on User Experience
Personalization significantly impacts user experience. When an app anticipates user needs and delivers relevant content, users feel understood and valued. This feeling of being understood leads to increased user engagement and satisfaction. Personalized recommendations, tailored interfaces, and relevant notifications enhance the overall user experience.
Designing Engaging and Relevant Experiences
Designing personalized experiences requires a thoughtful approach. Understanding user behavior and preferences is crucial. Data analysis should reveal patterns and trends to identify specific user segments. For instance, if a user frequently interacts with a particular game feature, the app could suggest similar features or content. Beyond simply remembering past actions, consider proactively suggesting items or content that align with the user’s profile and inferred interests.
Balancing Personalization and Privacy
User privacy is paramount. Personalization relies on user data, so transparency and clear data usage policies are essential. Users need to understand how their data is collected, used, and protected. Provide users with choices regarding data sharing and access. Implement strong security measures to protect user data from unauthorized access. Consider using anonymized or aggregated data to analyze trends and patterns without compromising individual user privacy.
Testing and Evaluating Personalization Features
Effective testing is key to gauging the success of personalization efforts. Track key metrics like user engagement, app usage time, and conversion rates before and after implementing personalization features. A/B testing is a valuable tool. Compare user responses to different personalization strategies to identify which methods are most effective. Use feedback mechanisms, such as surveys or app reviews, to gain insights into user perceptions of personalization. Focus groups can provide valuable feedback on the impact of personalization on user experience. For instance, track how often users return to the app after using a personalized recommendation.
Mobile App Personalization Strategies
Personalization in mobile apps isn’t just a nice-to-have; it’s a crucial element for driving user engagement and retention. Users expect experiences tailored to their needs and preferences. Smart personalization strategies help deliver relevant content, features, and offers, ultimately boosting app usage and satisfaction.
Behavioral Targeting
Behavioral targeting analyzes user actions within the app to understand their preferences and habits. This involves tracking things like app usage patterns, feature interactions, and content consumption. For instance, if a user frequently interacts with the “travel planning” section, the app can recommend relevant travel deals or articles. By understanding how users engage with the app, developers can deliver personalized recommendations that increase the likelihood of the user continuing their interaction with the app.
Contextual Personalization
Contextual personalization tailors the app’s experience based on the user’s current situation. Factors like location, time of day, or device type influence the content and features presented. Imagine a weather app dynamically adjusting its display based on the user’s current location, or a fitness app recommending workouts suitable for the time of day. This dynamic adaptation ensures the app is always relevant to the user’s immediate needs and context.
Predictive Modeling
Predictive modeling goes beyond analyzing current behavior by anticipating future user needs and preferences. Algorithms use historical data to predict what users might want or need next. This allows for proactive recommendations, such as suggesting a particular product or service based on past purchases or interactions. For example, a music streaming app might predict a user’s interest in a new artist based on their listening history and suggest relevant songs or playlists.
User Segmentation for Effective Personalization
Effective personalization strategies heavily rely on user segmentation. Dividing users into distinct groups based on shared characteristics allows for tailored experiences for each segment. This approach ensures that the personalization strategies are effective by targeting the specific needs and preferences of each user group. For example, a news app might segment users by their political leanings or preferred news categories, providing a more relevant and engaging experience.
Comparing Personalization Strategies Across App Categories
App Category | Behavioral Targeting | Contextual Personalization | Predictive Modeling |
---|---|---|---|
E-commerce | High – Recommending products based on browsing history. | Medium – Displaying location-specific deals. | High – Anticipating future purchase needs based on past behavior. |
Social Media | High – Suggesting relevant posts and connections. | Medium – Showing posts based on location and time. | High – Predicting future interests and suggesting connections. |
Finance | Medium – Providing personalized financial advice based on transaction history. | Medium – Offering location-specific financial tools. | High – Predicting future financial needs and suggesting appropriate investments. |
Travel | Medium – Recommending travel packages based on past searches. | High – Displaying location-specific travel deals and recommendations. | Medium – Predicting future travel interests and preferences. |
Note: The table above provides a general comparison. The effectiveness of each strategy can vary significantly depending on the specific app and its implementation.
Examples of Successful Personalization
Several companies effectively use personalization to enhance user retention and engagement. Netflix, for example, utilizes sophisticated algorithms to recommend movies and TV shows based on viewing history, genre preferences, and ratings. Spotify uses similar techniques to curate personalized playlists based on listening habits and preferences. These examples demonstrate the power of personalization to foster deeper user engagement and loyalty.
Challenges and Considerations
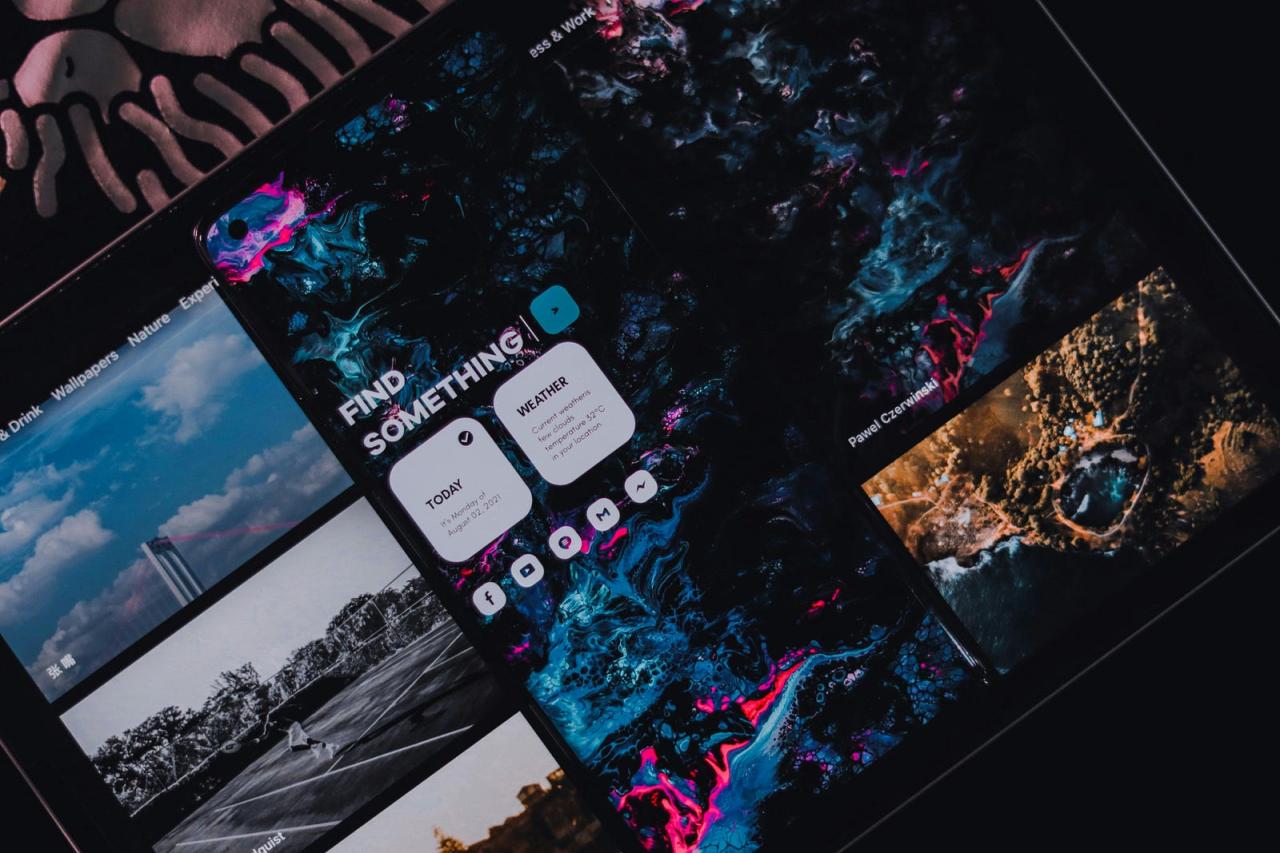
Personalization in mobile apps, while powerful, comes with its own set of hurdles. Ethical considerations, implementation snags, and potential negative impacts on user experience need careful attention. Successfully navigating these challenges is crucial for building trust and fostering a positive user relationship.
Implementing personalization requires a thoughtful approach that balances the benefits of tailored experiences with the rights and concerns of users. Careful planning and continuous monitoring are essential to ensure the ethical and effective use of personalization techniques.
Ethical Considerations: Data Privacy and User Consent
Data privacy and user consent are paramount in personalization. Users need to be informed about how their data is being used and have the option to opt out or control the level of personalization. This includes clear, concise explanations of data collection practices in the app’s privacy policy.
Transparency is key. Users should understand precisely what data is collected, how it’s used to personalize their experience, and what choices they have regarding this data. This proactive approach builds trust and fosters user confidence in the app.
Potential Implementation Issues
Several issues can arise during the implementation of personalization features. Data quality and quantity can significantly affect the accuracy and effectiveness of personalization algorithms. Insufficient data can lead to inaccurate predictions or limited personalization options.
Furthermore, technical complexities, like integrating with different data sources and ensuring seamless data flow, can create significant hurdles. Maintaining the performance and stability of the app while incorporating personalization logic requires careful engineering.
Addressing Negative Impacts on User Experience
Personalization, if not carefully implemented, can negatively impact user experience. Over-personalization, where recommendations become overwhelming or irrelevant, can lead to frustration and disengagement. A personalized experience should enhance user experience, not hinder it.
Finding the right balance is critical. Too much personalization can be intrusive, while too little can feel generic. User feedback is invaluable in this process, and features to allow users to adjust the level of personalization should be considered.
Importance of Ongoing Monitoring and Evaluation
Continuous monitoring and evaluation of personalization features are essential to maintain user trust and ensure continued effectiveness. Regularly assess the performance of personalization algorithms and their impact on user behavior and engagement.
This ongoing analysis should consider metrics like user satisfaction, app usage patterns, and the overall effectiveness of personalized recommendations. Regular reviews allow for adjustments and improvements based on real-time user data and feedback.
Future Trends in Mobile App Personalization
Mobile apps are evolving from basic tools to personalized companions. This shift is driven by a growing understanding of user needs and desires, pushing personalization beyond basic preferences to anticipate and proactively meet user expectations. The future of mobile app personalization is all about anticipating needs and providing experiences that feel intuitive and effortless.
AI and Machine Learning Powering Personalization
AI and machine learning are poised to revolutionize mobile app personalization. Sophisticated algorithms can analyze vast amounts of user data, including app usage patterns, interaction history, and even contextual information like location and time of day. This allows apps to predict user needs and tailor content, features, and recommendations in real-time. Imagine an app that anticipates you’ll need a certain type of information at a specific time based on past behavior, or a game that adjusts difficulty based on your skill level as it’s learned from your progress.
Personalized Notifications and In-App Experiences
Personalized notifications are moving beyond simple alerts to become more integrated into the overall user experience. By understanding user behavior and preferences, apps can deliver highly relevant notifications at the right time, increasing engagement and reducing the likelihood of users feeling overwhelmed by irrelevant messages. Imagine a fitness app subtly prompting you to take a short walk during a lull in your schedule, or an e-commerce app suggesting complementary products based on past purchases and browsing history. In-app experiences can be customized in a similar manner, offering tailored content, navigation, and interactive elements.
Hyper-Personalized Recommendations
Moving beyond simple recommendations, future personalization will focus on delivering hyper-personalized recommendations. This goes beyond suggesting similar products or content; it leverages advanced algorithms to identify unique patterns and predict individual needs. For instance, a music app could recommend artists that align with the user’s current mood as determined by listening history and real-time emotional data, not just based on genre. This level of personalization requires sophisticated data analysis and a deep understanding of user preferences.
Anticipated Future Impact on the Mobile App Industry
Aspect | Anticipated Impact |
---|---|
User Engagement | Increased user engagement and satisfaction due to a more tailored and relevant experience. |
App Retention | Improved app retention rates as users find the app more valuable and satisfying. |
Revenue Generation | Potential for increased revenue through targeted promotions, in-app purchases, and premium subscriptions. |
Competitive Advantage | Apps that effectively leverage personalization will gain a significant competitive edge. |
Data Privacy Concerns | Greater emphasis on user privacy and data security as users become more aware of the data being collected and how it is used. |
Illustrative Examples
Personalization in mobile apps isn’t just a fancy tech trick; it’s about tailoring the experience to each user. This section dives into practical examples, showing how to integrate personalization features effectively and responsibly. We’ll explore a hypothetical app and demonstrate how personalized recommendations, content, and user interactions can be implemented.
Let’s imagine a mobile app called “FoodieFind,” a restaurant discovery and review platform. We’ll illustrate how personalization can enhance the user experience, from recommendation engines to tailored content, all while respecting user privacy.
Hypothetical App: FoodieFind
FoodieFind aims to make restaurant exploration easier and more enjoyable. It allows users to search for restaurants based on location, cuisine, price, and reviews. Users can also save their favorite restaurants and create custom meal plans.
Personalized Recommendations
FoodieFind’s recommendation engine leverages user data to suggest restaurants that match their preferences. For example, if a user frequently searches for Italian restaurants in the downtown area and consistently leaves positive reviews, FoodieFind can suggest similar restaurants in the same area.
The app could also analyze a user’s past orders and dietary restrictions to recommend suitable options. A user who regularly selects vegetarian dishes and avoids gluten might see recommendations specifically tailored to those preferences.
The UI/UX for these recommendations needs careful consideration. The recommendations should be prominently displayed, perhaps in a dedicated “Personalized Recommendations” section or integrated seamlessly into the search results. Visually appealing thumbnails of restaurants, along with concise summaries of their attributes, will improve user engagement. Clear calls to action, like “View Menu” or “Save to Favorites,” are also important.
Data Privacy Considerations
Protecting user data is paramount. FoodieFind will employ robust security measures, encrypting data both in transit and at rest. Users will be provided with clear and concise privacy policies, detailing how their data is collected, used, and protected. They will have granular control over their data, allowing them to adjust their preferences and access, correct, or delete their information. Opt-in mechanisms are essential for collecting data, respecting user autonomy.
Examples of Personalized Content and Interactions
- Personalized Restaurant Reviews: FoodieFind can highlight reviews from users with similar tastes or dietary restrictions. This provides users with more relevant and insightful feedback.
- Customized Meal Plans: Based on a user’s preferences and dietary needs, FoodieFind can create personalized meal plans for a week, featuring restaurants and dishes that align with their choices.
- Location-Based Notifications: If a user frequently visits a specific neighborhood, FoodieFind can send notifications about new restaurants opening in that area or special promotions.
- Personalized Restaurant Profiles: The app can display tailored restaurant profiles, highlighting dishes, ambiance, and reviews most relevant to the user’s preferences. For instance, a user who loves live music might see a section in the restaurant profile dedicated to the music they offer.
FoodieFind’s success hinges on its ability to provide a personalized experience that respects user privacy. Implementing these features requires careful planning, attention to detail, and a commitment to data security.
Wrap-Up
In conclusion, mobile app personalization is not just a trend, but a crucial element for success in the app ecosystem. By understanding the nuances of data collection, implementation strategies, and ethical considerations, developers can build applications that foster stronger user engagement and loyalty. The future promises even more sophisticated personalization techniques, further enhancing user experiences.